Create step-changes in statistical methods to underpin R&D in sustainable primary industries
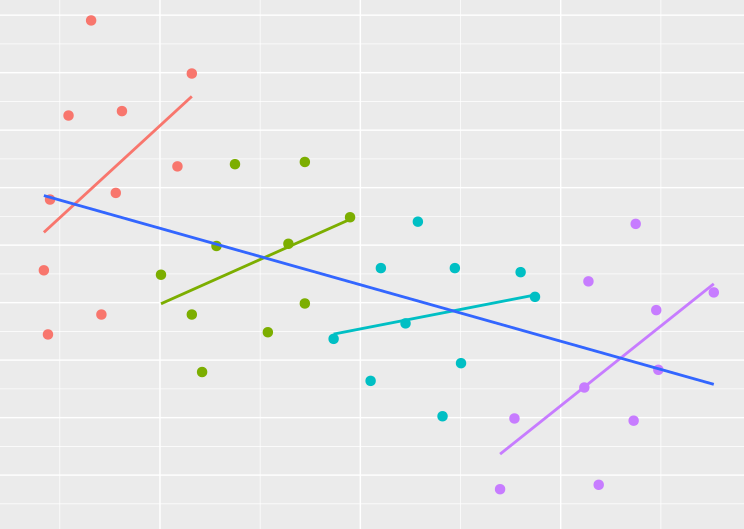
Create step-changes in statistical methods to underpin R&D in sustainable primary industries
Our people are statistical scientists who collaborate with industries and research providors in primary industries to develop statistical methods which have a measurable impact on the reliability of information obtained from the conduct of designed experiments. This in turn increases the confidence of stakeholders in this information and technologies which facilitates more rapid and widespread adoption.
The major focus of our work centres on plant improvement where our methods and software tools enable breeders to meet the challenges of improving productivity at the farm-gate in the face of rapidly changing environment and market scope.
MMaED has collaborated with 13 industry researchers.
MMaED has produced and collaborated on 6 statistical software solutions: Pedicure, ODW, DWReml, dwrPlus, ASRextras, ASReml-R
MMaED has published 400+ scientific publications, which is a testament to our remarkable dedication and invaluable contributions to the of utilization of linear and generalised linear mixed models, with particular application to plant and animal breeding data.
With over four decades of experience, MMaED is at the forefront of utilizing mixed models and experimental design. We excel in improving statistical methodologies in the realm of plant and animal breeding data analysis, facilitating sustainable advancements in genetics.
DWReml is an R package that fits the general linear mixed model and estimates variance components by Residual Maximum Likelihood using the Average Information algorithm. DWRreml exploits a publicly available open source supernodal sparse linear equation solver (MUMPS: MUltifrontal Massively Parallel sparse direct Solver) to efficiently solve the mixed model equations. The package offers a functional style user interface supporting several common variance models and allows partitioning of the (possibly correlated) experimental units into independent sections.
ODW is an R package to generate optimal categorical experimental designs under a general linear mixed model. Given an initial configuration and an objective set of effects, ODW iteratively interchanges rows (experimental units) of the corresponding column partition of the incidence matrix in search of a permutation that minimizes the A-value for the objective effects. The package offers a functional style user interface similar to DWReml and a concise set of variance models.
Phone: +61 2 4221 5641
Email: brian_cullis@uow.edu.au
Location: Building 39C Room 266
I am honoured to lead such a passionate, innovative and dedicated team of statistical scientists. Each member of the team make a difference each and every day with their commitment to providing relevant and bespoke statistical solutions to solve problems for the primary industries R&D sector.
Senior Professor Brian Cullis
CBADS-SPI Director and Senior Professor
Email: brian_cullis@uow.edu.au
Dr David Butler
Principal Research Fellow
Email: david_butler@uow.edu.au
Dr Alison Smith
Principal Research Fellow
Email: alison_smith@uow.edu.au
Dr Beverly Gogel
Senior Research Fellow
Email: beverly_gogel@uow.edu.au
Aidan McGarty
Associate Research Fellow
Email: aidan_mcgarty@uow.edu.au
Lu Wang
Associate Research Fellow
Email: lu_wang@uow.edu.au
Dr Chris Lisle
Honorary Research Fellow
Email: chris_lisle@uow.edu.au
Dr Luke Mazur
Honorary Research Fellow
Email: luke_mazur@uow.edu.au